How to predict customer churn
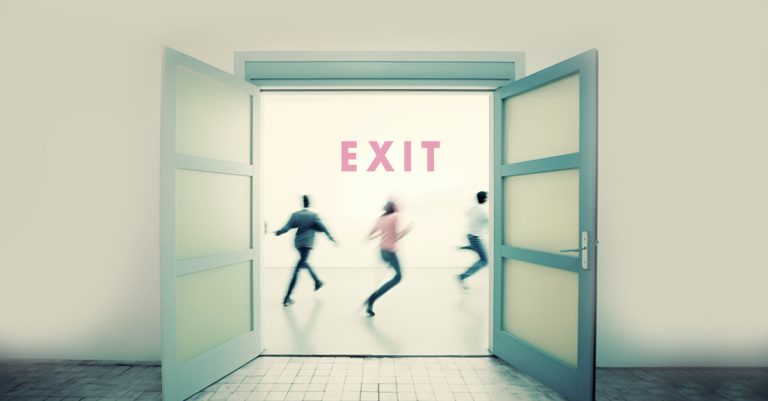
- marketing
- November 7, 2022
- Categories: Artificial Intelligence
- Tags: Customer Churn
What is Churn?
Churn is the state in which individuals or items move out of a collective group, as a brand. It is one of two primary factors that determine the steady-state level of customers a business will support.
Customer churn is a business metric that measures the number of customers, accounts, etc. that a business has lost over some time and is typically expressed as a rate, being the inverse of the renewal rate, i.e. an 80% renewal rate is the equivalent of a 20% customer churn rate.
How does Churn occur?
Many factors can be influencing the reasons for a customer to churn, such as competition or maybe poor service, so one can never be so sure; from the side of data science, patterns in the provided data are looked upon so that they could be identified during data analysis.
Customer satisfaction, happiness, and loyalty can be achieved to a certain degree, but churn will always be a part of the business. Churn can happen because of:
- Bad customer service (poor service quality, response rate, or overall customer experience),
- Financial issues (fees and rates),
- Competition,
- Long-time customers don’t feel appreciated
What is Churn Prediction?
Churn Prediction focuses on identifying these patterns through data analysis so that it could predict which customers are most likely to leave a company based on their usage of the service.
From a company point of view, this is very critical information, because acquiring new customers is much more costly than retaining the existing ones and this is why the insights gained from Churn Prediction help them to concentrate more on the customers that are at a high risk of leaving.
The output of Churn prediction is a simple yes or a no, predicting 1 if the customer is likely to churn and 0 if otherwise.
To wrap it all up, as the primary function of churn prediction is to predict a list of potential churners so that providers can start targeting them by retention campaigns, it becomes a huge advantage for a company, as well as a potential money saver, especially for companies with large customer acquisition costs.
Churn classification
Software as a service (SaaS) with its subscription-based business model and membership-based businesses are at the forefront of innovative customer retention strategies. Analyzing growth in this space might involve tracking metrics (like revenues, new customer ratio, etc.), performing customer segmentation analysis, and predicting lifetime value.
The churn rate is an input of customer lifetime value modeling that guides the estimation of net profit contributed to the whole future relationship with a customer. Independently, it calculates the percentage of discontinuity in subscriptions by customers of a service or product within a given time frame.
This translates to revenue loss via customer cancellation. Market saturation is quite evident in the SaaS market, there are always plenty of alternatives for any SaaS product. Studying the churn rate can help with Knowing-Your-Customer (KYC) and effective retention and marketing strategies for subscription-driven businesses.
You can classify churn as:
- Customer and revenue churn
- Voluntary and involuntary churn
Customer and revenue churn: Customer churn is simply the rate at which customers cancel their subscriptions, while revenue churn is the loss in your monthly recurring revenue (MRR) at the beginning of the month. Customer churn and revenue churn aren’t always the same. You might have no customer churn, but still have revenue churn if customers are downgrading subscriptions.
Voluntary and involuntary Churn: Voluntary churn is when the customer decides to cancel and takes the necessary steps to exit the service, while Involuntary churn happens due to situations such as expired payment details, server errors, insufficient funds, and other unpredictable predicaments.
The challenges of building a churn model
The impact of the churn rate is clear, so we need strategies to reduce it. Predicting churn is a good way to create proactive marketing campaigns targeted at customers about to churn.
Thanks to big data, forecasting customer churn with the help of machine learning is possible. Machine learning and data analysis are powerful ways to identify and predict churn. During churn prediction, you’re also:
- Identifying at-risk customers,
- Identifying customer pain points,
- Identifying strategies/methods to lower churn and increase customer retention.
Here are the main challenges that might make it difficult for you to build an effective churn model:
- Inaccurate or unstructured customer data,
- Lack of information and expertise,
- Incoherent churn modeling approach,
- Right metrics to validate churn model performance,
- Churn event censorship
Churn Prediction in Machine Learning
When it comes to using business applications of machine learning, it doesn’t get much better than customer churn prediction. It’s a problem where you usually have a lot of high-quality, fresh data to work with, it’s relatively straightforward, and solving it can be a great way to increase profits, as churn is, amongst others, a good indicator of growth potential.
Churn rates track lost customers, and growth rates track new customers—comparing and analyzing both of these metrics tells you exactly how much your business is growing over time. You can say your business is growing if growth is higher than churn. If churn is higher than growth, your business is getting smaller.
Churn prediction workflow
The overall scope to build an ML-powered application to forecast customer churn is generic to a standardized ML project structure that includes the following steps:
- Define the problem and goal, understanding the right insights from the analysis and prediction. collecting requirements, stakeholder pain points, and overall expectations.
- Data source: Specify the necessary data sources for the modeling stage. Some popular sources of churn data are CRM systems, analytics services, and customer feedback.
- Data preparation, exploration, and preprocessing: Raw historical data for solving the problem and building predictive models need to be transformed into a format suitable for machine learning algorithms. This step can also improve overall results by increasing the quality of data.
- Modeling and testing: This covers the development and performance validation of customer churn prediction models with various machine learning algorithms.
- Deployment and monitoring: This is the last stage in applying machine learning for churn rate prediction. Here, the most suitable model is sent into production. It can be either integrated into existing software or become the core of a newly built application.